Talk Details
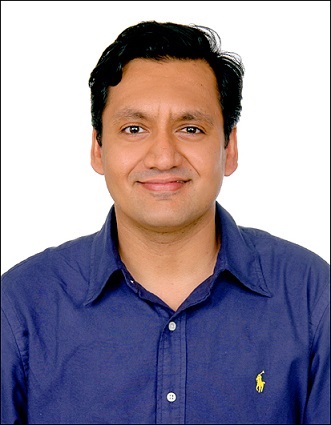
Fairness vs Efficiency, by Siddharth Barman
Algorithms are being used increasingly as decision making tools in socially sensitive settings, such as criminal justice, medical testing, and online advertising. The bias of such algorithms (and the resulting discrimination in their predictions) is a serious concern that motivates the study of fairness in AI systems. Recent studies show that enforcing fairness guarantees, by post hoc adjustments, can end up adversely effecting the performance/accuracy of the underlying system.
In this talk, I will outline our efforts which establish that such an extreme tradeoff between fairness and accuracy is mitigated in relevant decision-making settings. Specifically, we focus on settings in which decisions have to be made by applying the same threshold on every individual's outcome probability. Within such contexts, we formulate fairness as a classification performance measure---in particular, as accuracy with respect to fair labels---and prove that accuracy and fairness can be achieved together.
Based on joint work with Arpita Biswas, Amit Deshpande, and Amit Sharma.
About the speaker
Siddharth Barman is an assistant professor in the Department of Computer Science and Automation at the Indian Institute of Science. Before joining IISc, he was a post-doctoral scholar at Caltech and obtained his Ph.D. in Computer Science at the University of Wisconsin-Madison. Siddharth is a Ramanujan Fellow and his research lies at the interface of Computer Science and Microeconomics. His work spans multiple areas including Approximation Algorithms, Game Theory, and Machine Learning. Siddharth’s current work addresses fairness in the context of algorithmic decision making. Here, a specific goal of his research is to quantify tradeoffs between efficiency and fairness.